국제 음향 음성 신호처리 학술대회인 IEEE ICASSP 2021에 Motion AI 팀의 Efficient Adversarial Audio Synthesis via Progressive Upsampling 연구가 소개되었습니다.
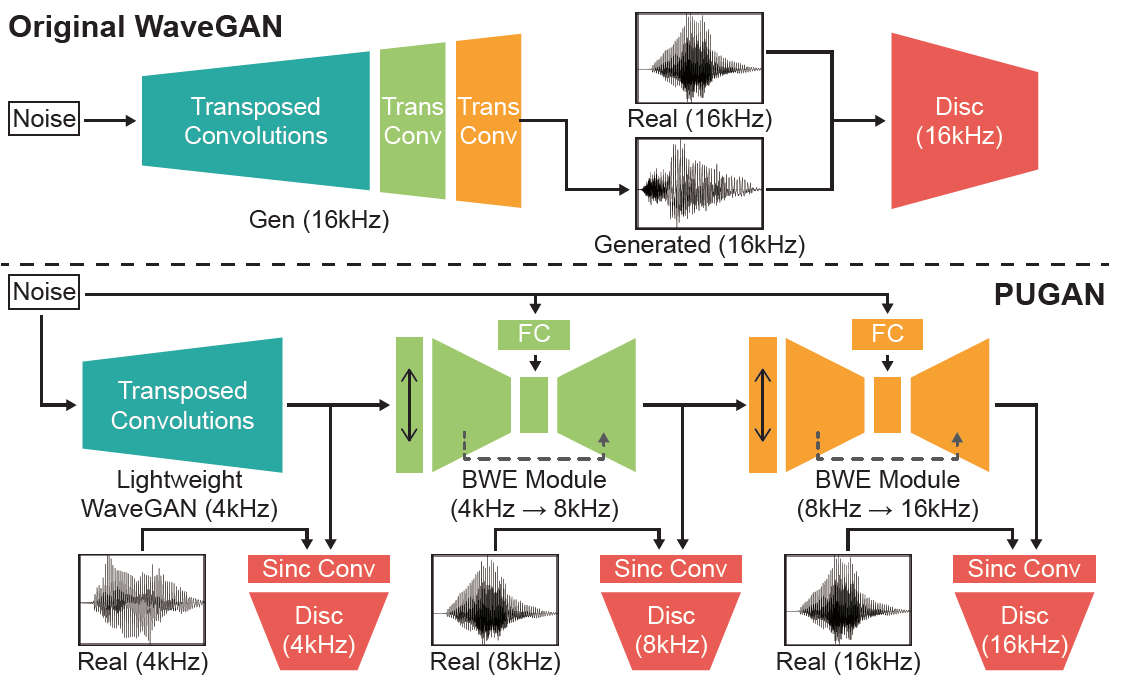
Abstract
This paper proposes a novel generative model called PUGAN, which progressively synthesizes high-quality audio in a raw waveform. Progressive upsampling GAN (PUGAN) leverages the progressive generation of higher-resolution output by stacking multiple encoder-decoder architectures. Compared to an existing state-of-the-art model called WaveGAN, which uses a single decoder architecture, our model generates audio signals and converts them to a higher resolution in a progressive manner, while using a significantly smaller number of parameters, e.g., 3.17x smaller for 16 kHz output, than WaveGAN. Our experiments show that the audio signals can be generated in real time with a comparable quality to that ofWaveGAN in terms of the inception scores and human perception.
Authors
Youngwoo Cho(KAIST), Minwook Chang(NCSOFT), Sanghyeon Lee(KAIST), Hyoungwoo Lee(KAIST), Gerard Jounghyun Kim(Korea University), and Jaegul Choo(KAIST)
Proceeding
IEEE International Conference on Acoustics, Speech and Signal Processing 2021(ICASSP)